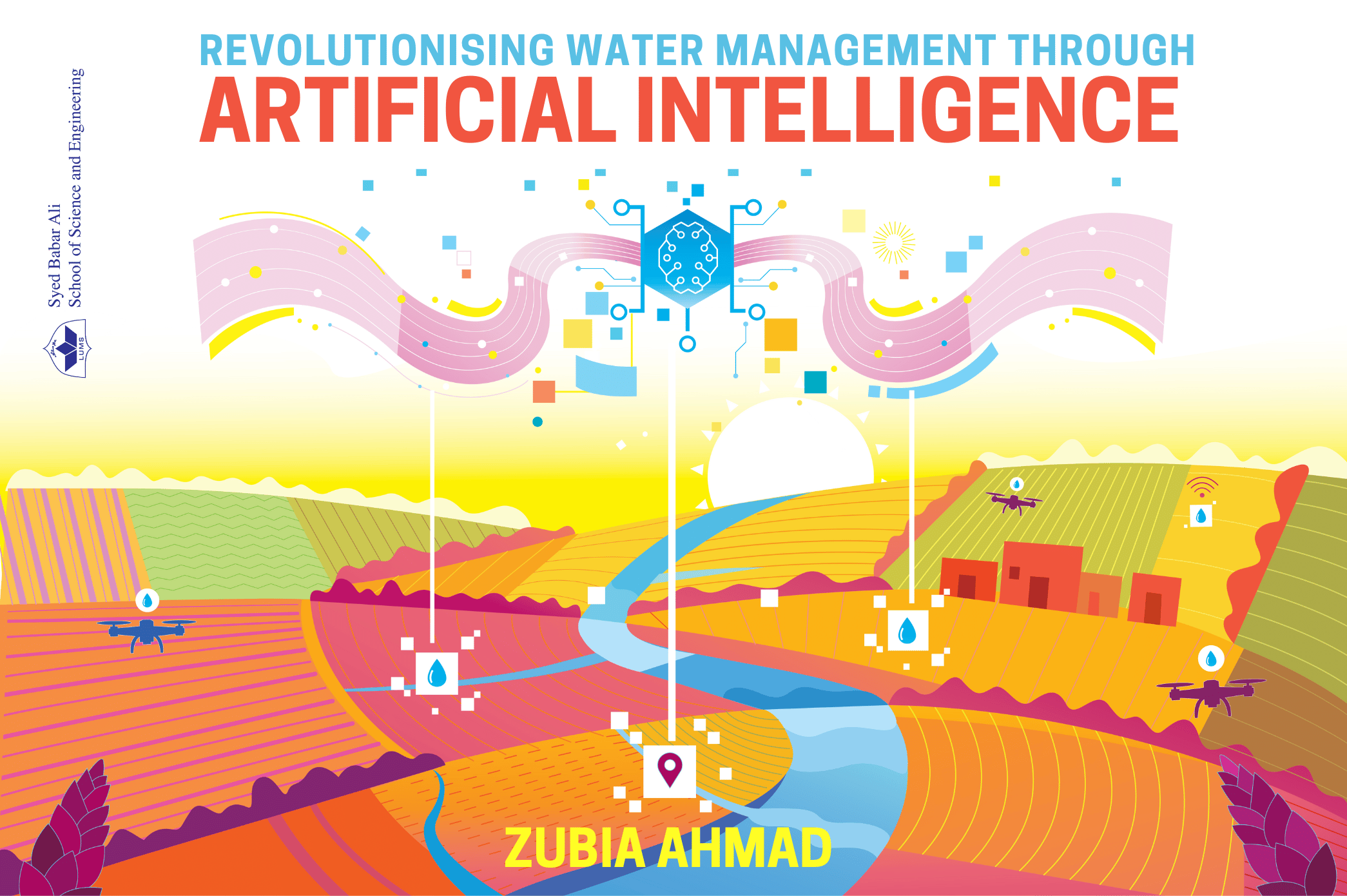
Revolutionising Water Management through Artificial Intelligence
This story was originally published in Particle 21.
It was a monumental ship that we thought was indestructible, and what we found out was that we are still vulnerable to forces on this planet that are beyond our control,”
Remarked Jules Jaffe, a research oceanographer at the Scripps Institution of Oceanography at the University of California, who helped find the Titanic wreckage in 1985.
From the sinking of the Titanic in 1912 to the recent demise of the Titan submersible during its expedition to explore the ship’s remains in 2023, we have witnessed the unpredictable and formidable nature of water in various manifestations, whether in the depths of the ocean, flooding on land, or even its abundance. Water, a life-giving resource, exhibits a force beyond human control.
In the same vein, Artificial Intelligence (AI) has emerged as another seemingly elusive force. The launch of chatGPT, an AI chatbot by OpenAI, has redefined the standards of AI, demonstrating machines’ remarkable ability to “learn” and comprehend the intricacies of human language and interaction. However, the rapid advancements in AI technologies have raised concerns about potential risks to society and humanity. Shortly after chatGPT’s release, prominent figures in the tech industry, such as Elon Mask, called for a 6-month temporary pause on the rapid developments of advanced AI technologies.

Nevertheless, researchers at LUMS have harnessed the power of AI for water management in agriculture. To enhance water distribution to crops, the NCRA-Agriculture and Robotics Lab (NARL) has collaborated with the Punjab Irrigation Department (PID) in a project supported by the Asian Development Bank (ADB). Led by Dr. Abubakr Muhammad, Dr. Murtaza Taj and Dr. Hassan Jaleel. The collaborative effort utilised earth observation data to improve the functioning and distributary canals in the Lower Bari Doab Canal (LBDC).
Dr. Murtaza Taj and his team member Dr. Usman Nazir focused their efforts on the crop classification aspect that provided critical input to the decision-making tool.
The outcome was the development of an equitable water distribution system that provides sufficient water to different areas according to the crops’ requirements. However, classifying crops based on multispectral imagery poses significant challenges. This is where AI steps in, offering valuable solutions.
Using spatiotemporal analysis from the Copernicus Sentinel 2 satellite imagery and employing machine learning algorithms, the researchers successfully identified the crops. As the name suggests, the beauty of machine learning is its ability to develop algorithms and models that enable computers to learn and make decisions based on input data.
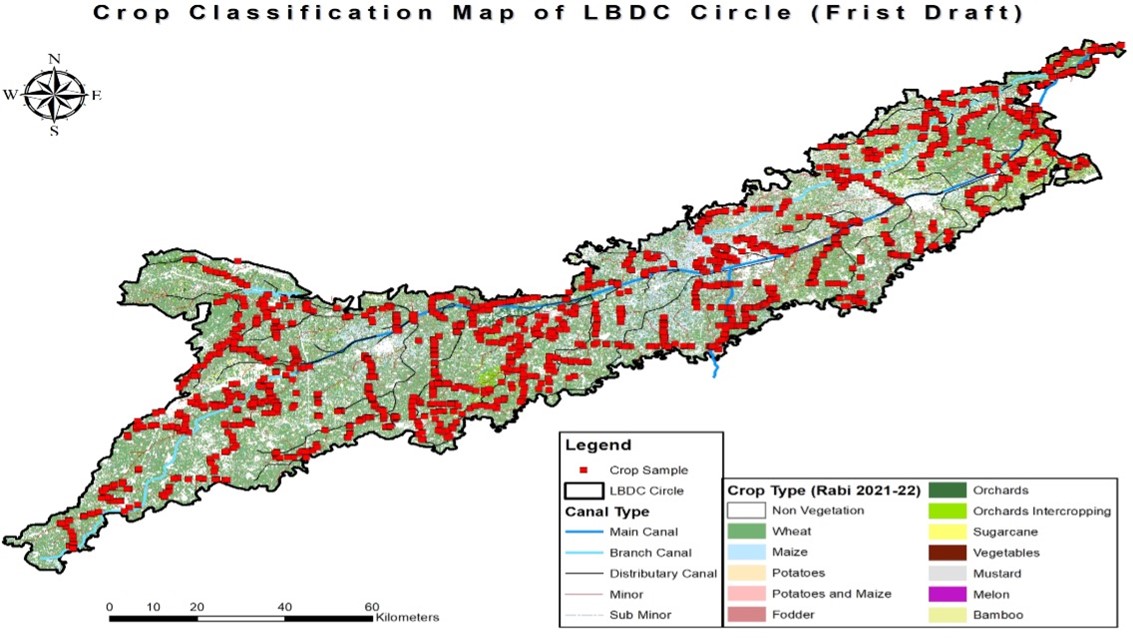
To achieve crop classification, Dr Murtaza and his team initially devised a unique architecture that combines convolutional neural networks (CNNs) for spatiotemporal analysis. Inspired by the organisation and functioning of the visual cortex in the human brain, CNNs are specifically designed for processing and analysing visual data like images and videos. This approach allowed them meaningful data and achieve higher accuracy compared to traditional methods and previous deep learning approaches, like Random Forest (RF). Their methodology involves usage of 3D CNNs to capture spatiotemporal patterns and 1D CNNs for redefined temporal analysis of the image data. This combined approach significantly improves crop classification accuracy by 2% while keeping the computational complexity (such as time and memory) low. However, it’s important to note that CNNs are powerful algorithms that require substantial computational power and memory resources to process data in three dimensions.
Known for its efficiency and scalability, Random Forest on the other, can handle crop classification when the data does not have complex spatial and temporal dependencies. The lower computational power required by RF makes it more practice for implementation by the PID, thus driving its adaptation over CNN. This machine-learning technique combines multiple decision trees to make predictions, with each tree offering its own prediction.
The Random Forest algorithm follows a series of steps to make predictions. First, it collects relevant data related to the problem. In this case, the PID collected two types of training data, point samples and polygon samples, for thirteen crops from the LBDC region. Next, the algorithm creates multiple decision trees, each focusing on different features of the data. The final prediction is determined by considering the input from all the trees. It’s akin to having a panel of experts, each offering their opinion, and the final decision is reached by considering everyone’s input. This "wisdom of the crowd" approach ensures more accurate and reliable results. Once trained on existing data, the Random Forest can use its collective knowledge to make predictions on new, unseen data, making it a versatile and powerful tool for various applications.
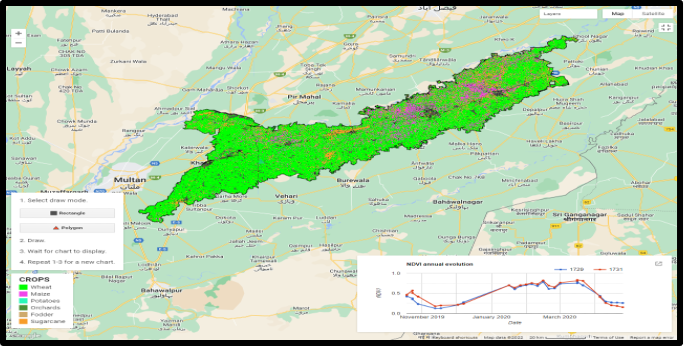
To further enhance the accuracy of Random Forest, Dr Murtaza and Dr Usman implemented a masking mechanism to filter out built-up regions. Using the samples provided by PID, the researchers trained and tested a multi-spectral classifier for crop classification, archiving an impressive accuracy of 96,78%.
This crop classification algorithm has become an integral part of PID’s Decision Support Systems (DSS) within LBDC. The DSS aims to forecast water demand for each 8-day period and communicate it to the Indus River System Authority (IRSA) for diversion determination.
By supplying water based on the specific crop type and age, the system not only closes the supply-demand gap but also prevents over-irrigation and crop water stress, thus maximising crop return per unit of water.
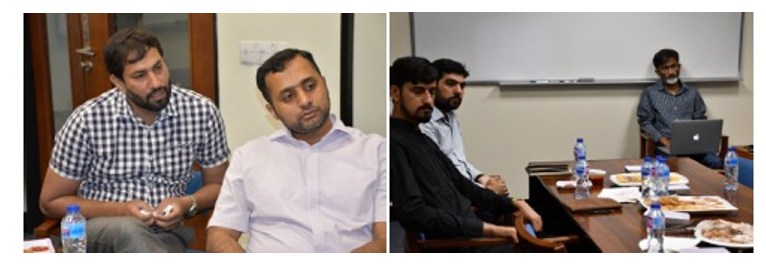
From Canal to Flood— utilising AI to Understand Disaster-Stricken Districts
Once the Random Forest algorithm was trained based on crop samples collected by the Punjab Irrigation Department (PID), its application expanded to any area with similar crops, even those struck by floods.
Immediate relief and rescue operations are the initial response to flooding, but the impact of such natural disasters extends far beyond the immediate aftermath. In August 2022, heavy rainfall in the southern region of Pakistan led to severe flooding, resulting in significant damage to agricultural land and the destruction of standing crops.
As part of ongoing research, the NARC-Agriculture and Robotics Lab (NARL) and Center for Water Informatics & Technology (WIT) at LUMS have collaborated with the NASA HARVEST team at the University of Maryland to analyse the wide-ranging effects of the 2022 floods on the winter (Rabi) crop. The researchers assessed the crop damage by comparing pre-and post-flood satellite-based remote sensing data, mapping the flooded areas and classifying crops in the flood-stricken districts of Southern Punjab, Sindh and Baluchistan in 2022.
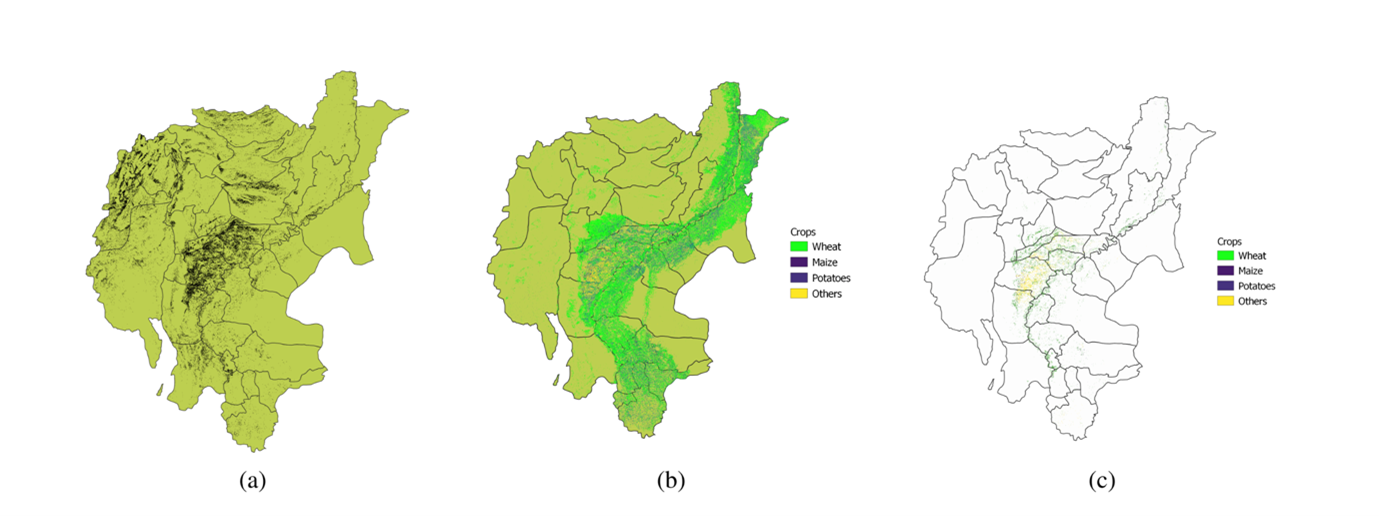
Another factor contributing to a shortage of agricultural produce after flooding is the highly saturated soil, which may not be suitable for sowing. The researchers analysed a satellite-based soil moisture product (SMAP Level-4) to determine the changes in the soil moisture content before the flooding event and after the receding of floodwater. This analysis allows researchers to determine when the soil will be ready for sowing and thus predict food security.
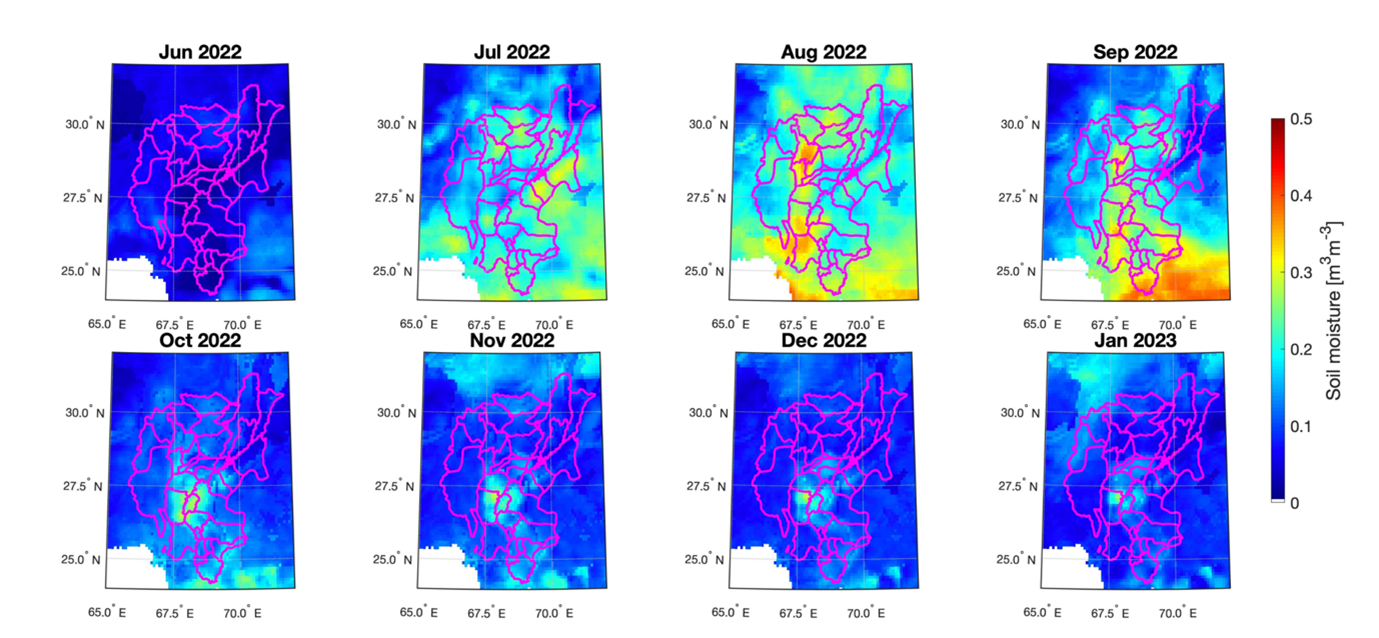
Water Sense – A Ground-level Soil Moisture Sensor
Monitoring soil moisture is crucial not only during periods of water influx but also during times of abundance. With Pakistan predicted to face water scarcity by 2025, where the agricultural sector consumes 90% of freshwater resources, accurate soil moisture monitoring becomes even more essential. To address this need, the Center of Water Informatics & Technology (WIT), in collaboration with Nestle’s Pakistan’s Caring for Water Initiative, developed Water Sense, an Internet of Things (IoT) powered soil moisture sensor. Water Sense operates on the capacity measurements principle, ensuring precise and durable reading without hurting the soil chemistry. The main objective of these sensors is to conserve up to 50% of irrigation water by empowering farmers to make informed irrigation decisions.
In a pilot project conducted in 2021, WIT installed 107 Water Sense sensors across Punjab for Nestle Pakistan. Based on the data from maize crops, an average irrigation savings of 25% was reported, with no apparent yield penalty. This amounts to net water conservation of 142 million litres from the 178 acres of the Maize crop (0.8 million litres per acre), using 38 soil moisture sensors (3.7 million liters per sensor).
Compared to other efficient irrigation systems like drip irrigation and rail gun, Water Sense provides a cost-effective option due to its capital expense. The affordability makes it an ideal choice, even for smaller agricultural lands. Considering the significance of water conservation, every drop counts.
WIT is further expanding its research on Water Sense, incorporating it into a larger framework that will utilise AI (machine learning algorithms) with ground data and satellite observations to measure soil moisture. Dr Abu Bakr Muhammad, the founding director of WIT and associate professor at the Department of Electrical Engineering, has received the prestigious Khalid and Mussarrat Aziz research grant for this initiative.
The fusion of technology and water management is paving the way for a more resilient and productive agriculture sector, offering hope for a future where humans can better understand and ensure food security in the face of climate change and increasing water scarcity.
Water Desalination: A solution to Water Scarcity?
Despite covering 71% of the Earth’s surface, the amount of water available for human use and consumption is limited. Water desalination, the removal of salt and other impurities from seawater or brackish water, offers a potential solution. At LUMS, the Laboratory of Advanced Membranes and Separations (LAMS) have used recycled plastic bottles to fabricate membranes for desalination. Learn more about the research on Water Desalination here.
The LUMS Agricultural Land
Other than labs, the SBASSE has provided dedicated agricultural land on campus for researchers to conduct innovative studies. These initiatives drive advancement in the field of agriculture and tackle grand challenges of ensuring a sustainable supply of energy, food and water.
For instance, WIT utilises these grounds to test and refine their soil moisture devices, improving their effectiveness in monitoring soil conditions. While Dr Khurram Bashir, a renowned plant biologist at LUMS studies ethanol’s use to enhance drought tolerance in crops like wheat and rice. This ground-breaking research paves the way for improved crop development in drought-prone areas. You can learn more about Dr Khurram’s research here.
Dr Nauman Zafar Butt from the Department of Electrical Engineering is also utilising the LUMS agricultural for his argivoltaic research, which involves monitoring the impact of solar panels on crops. This initiative is the second recipient of the prestigious Khalid and Mussarat Aziz Research Grant for Planet Earth.
Another noteworthy research revolving around the roots of plants at LUMS, is Dr Zaigham Shahzad’s study on improving phosphorus utilisation in rice plants. This research has also received a prestigious grant, and you can find more information about it here.