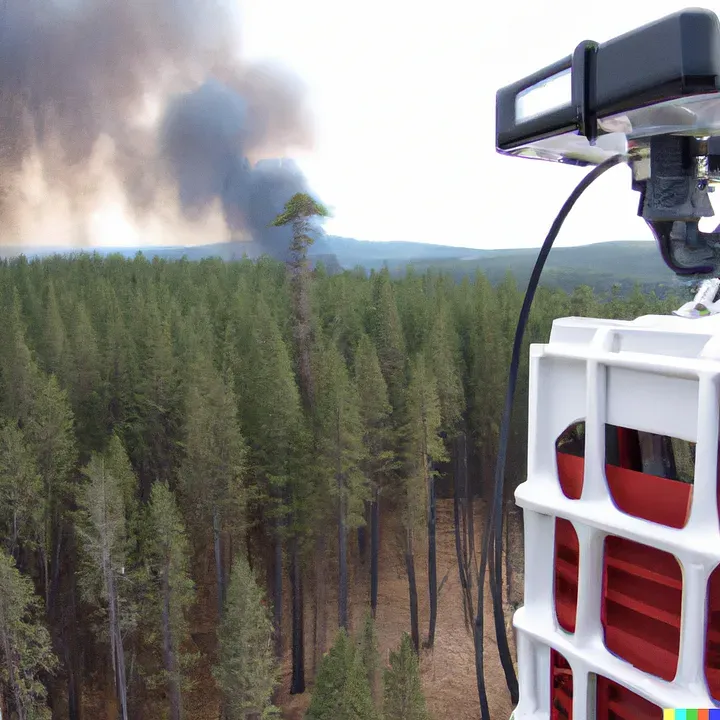
Exploring Technology-Driven Approaches for Early Detection and Response to Forest Fires: The LUMS Perspective
This story was originally published in Medium
As our partners, WWF-Pakistan highlighted in their initial blog post about this pilot, Pakistan is among the most vulnerable countries to the effects of climate change. The climate anomaly was so far most visible in the high mountain regions in the northern regions of Pakistan, where glaciers were melting at an increased pace, resulting in disasters like, i.e. rain-fed flash floods and Glacier Lake Outburst Floods (GLOF). But the year 2022 can be considered unprecedented in this regard as the country was first hit by an extreme heatwave that culminated in the worst-ever monsoon floods in the Indus River basin.
Solving grand challenges that face the global community needs a collaborative approach and interdisciplinary knowledge base. Researchers at Syed Babar Ali School of Science and Engineering (SBASSE), Lahore University of Management Sciences (LUMS) actively engage in multidisciplinary research and stakeholder engagement to understand and solve these grand challenges. From saving forests to saving wildlife, engineers at SBASSE are making interventions at a range of grand challenges with a diverse group of stakeholders.
When people from different disciplines and experiences come together, new ideas are often born. That’s exactly what happened during a visit by the WWF team to the NCRA-Agricultural Robotics Lab (NARL) at SBASSE, LUMS, earlier this year. In the context of increased forest fire events in Pakistan during the summer of 2022, the WWF team and the Lab brainstormed technology-enabled solutions for the early detection of forest fires. The Lab and WWF-Pakistan had previously collaborated on similar projects at the intersection of ecology and technology, such as a predator early warning system. The team believed that if a technology-enabled fire detection system detects a fire before it spreads, it can help reduce the risk to life and limb through a timely and coordinated response. With the help of our project champions at the UK Foreign, Commonwealth and Development office (FCDO), this idea became the current Frontier Technology Livestreaming pilot.
NCRA-Agricultural Robotics Lab (NARL) is a constituent lab of the National Centre of Robotics and Automation (NCRA), a consortium of 11 labs across 13 universities in Pakistan, all working in the domain of robotics and Automation. NARL research focuses on efficient irrigation, sustainable digital agriculture, identifying pathways of water quality degradation, forest assets management, and other adjacent domains. The lab has capabilities in the domains of robotics, real-time IoT-based sensors, remote sensing, computer vision, and artificial intelligence (AI).
There are several ways that technology can help with forest fire mitigation and preparedness. For example, remote sensing technologies such as satellites and drones can be used to monitor the health of forests and detect potential fire risks. This can help firefighting teams to identify areas that are at high risk of forest fires and take action to prevent them. Another way that technology can help is by providing real-time information and updates about fires. This can include real-time detection and monitoring technologies that can help firefighting teams to respond more quickly and effectively to forest fires.
The LUMS team has extensive experience developing Early Warning Systems and high-level decision support systems. This includes real-time early warning systems against predator threats to communities living in the wilderness, real-time early warning systems to reduce the impact of disasters like flash floods, etc. An Early Warning System based on in-situ sensors integrated with remote sensing offers us the ability to scale the geographic scope of technology rapidly. An example is a decision support system irrigation management using remote sensing integrated with digital in-situ sensors. In another project, a team of researchers made an AI-powered vision-based system to monitor, classify and quantify trash in water bodies. The trash monitoring system consisted of Edge-AI-enabled IoT cameras that could easily be adapted to various use cases. We came up with the idea to leverage above mentioned capabilities in Artificial Intelligence (AI), Internet of Things (IoT), edge AI, remote-sensing, computer-vision, and our prior work in integrated decision support and early warning systems to this exciting and challenging use case.
Thus, we planned to adapt the AI camera-based predator early warning system for forest fire detection as a cost-effective and scalable solution. All it needs is training the AI models to detect the forest fire instead of a predator. The task is challenging due to the limited availability of relevant data sets, yet doable. The system was already designed for off-grid deployments at remote locations, a needed capability for forest fire detection. We also took inspiration from the irrigation management decision support system and planned to adapt it for fire hazard predictions. The irrigation management system develops scaled maps of the soil moisture requirement for crops using open-source satellite imagery. At the same time, a small network of Soil Moisture Sensors feeds real-time and accurate data for a real-time prediction map. A similar approach can be used to develop scaled forest fire risk maps using real-time IoT weather stations and open-source satellite imagery. Our prior experience working on climate change-related challenges not only helped us empathize with the problem, but we also had a better understanding of the technical and non-technical challenges that we could incorporate into the product design.
The Proposed Interventions
To come up with a technology-driven solution for the early detection and response to forest fires, the project team at LUMS explored the challenge holistically and conceived a solution set that had the potential to impact the challenge at the three stages of the disaster response:
● Mitigation
● Preparedness
● Response
The solution proposed by the LUMS team will be the first such innovation in Pakistan. Considering the country’s economic and infrastructural context, the scalability and cost-effectiveness of any proposed intervention take center stage. Thermal cameras are one solution that has been used for detecting forest fires because they can detect heat signatures, even at long distances, making them effective for spotting fires in the early stages before they become large and difficult to control. Thermal cameras can also be used to monitor the spread of a fire and track its movement, which can help firefighters to respond quickly and effectively. But this option was not feasible in our context due to the high capital cost and dependence on overseas vendors to ensure the upkeep and maintenance of the equipment.
Can low-cost tech be used to detect forest fires and build early warning systems? To answer this challenging question, we proposed multi-tier solutions that are expected to create a whole bigger than the sum of its parts:
- AI-Powered real-time IoT camera for fire detection and real-time monitoring
- Using remote sensing to create fire hazard risk maps and to predict the direction and speed of fire spread after the onset.
- IoT Automatic Weather Stations to calibrate remote sensing models, as well as provide real-time coverage during the disaster response
- Cost-effective IoT forest fire detection sensors as a parallel approach and also to increase coverage in high-risk areas.
AI-Powered real-time IoT camera is the key component for fire detection over a vast forest area. To ensure the solution’s cost-effectiveness and sustainability over the project’s lifecycle, we proposed deploying a commonly available pan-tilt-zoom RGB camera deployed on fire towers. Using AI to enhance these cameras’ capabilities, they can effectively detect the onset of a forest fire event without compromising the system’s scalability, as these RGB cameras are cost-effective and easier to maintain.
We also integrated satellite-based remote sensing into our proposed Early Warning System. Where the cameras help detect the onset of a fire, Satellite-based remote sensing can help in preparedness and mitigation activities. Satellite data can help create scaled maps of the forest to determine the soils and the forest fuel (combustible biomass found in forests), dryness, and many other parameters. A weekly or biweekly updated map of these parameters can help forest fire rangers to plan, strategize and prioritize their fire prevention measures. Satellite data can also help predict the direction and speed of the fire spread once it starts. We are exploring remote sensing algorithms for this use case as well.
Ground truthing is a crucial step to enhance the accuracy of Satellite-based remote sensing; thus, we also planned to deploy Automatic Weather Stations to collect real-time data. The IoT-enabled automatic weather stations send real-time data on essential weather parameters, i.e., temperature, humidity, wind speed, soil and combustible fuel moisture, solar radiation, etc. Open-source remote-sensing imagery options, though cost-effective, generally have a low spatial resolution (large size of a single pixel) and low temporal resolution (just 1–4 scans each month). The IoT automatic weather stations enable us to collect real-time ground truthing data for remote sensing calibration and fine-tune AI models for projected risk maps in the absence of the latest satellite imagery.
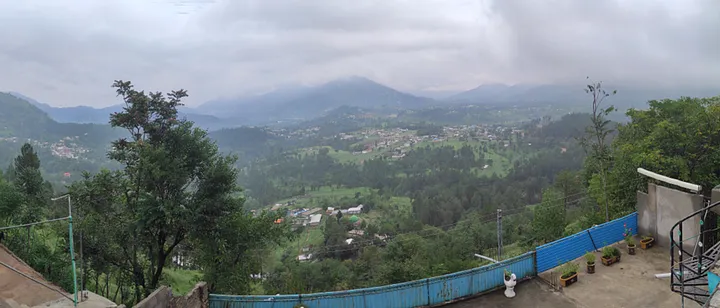
Another interesting innovation is using cost-effective IoT fire detection sensors to enhance the early warning system’s features. IoT sensors offer increased detection capability at a more granular range. A network of such sensors can be used for fire detection in a specified area. The lab has extensive experience deploying real-time Internet of Things (IoT) sensors at challenging locations with remote access and harsh weather, and we wish to leverage that experience for an impactful use case of the IoT ecosystem.
All these systems can potentially intervene in some stage of disaster response. Where the AI camera and IoT sensors help in early detection and thus better response, Remote sensing allows better preparedness and mitigation activities. With an integrated approach, we wish to develop an early warning system that is scalable as well as adaptable to different use cases and needs.
Overcoming Obstacles
The various solutions proposed by the LUMS team, although very innovative, are based on assumptions that are critical to the planned outcomes. For example, building AI for forest fire detection requires a lot of actual forest fire images to for training the AI model. We expected to utilize the commonly available data sets (forest fire images). But we found out that the available imagery data were mostly candid photos and were not useable from the point of view of a forest fire watchtower-mounted camera. We adapted and used simulated imagery from computer games 3D engines.
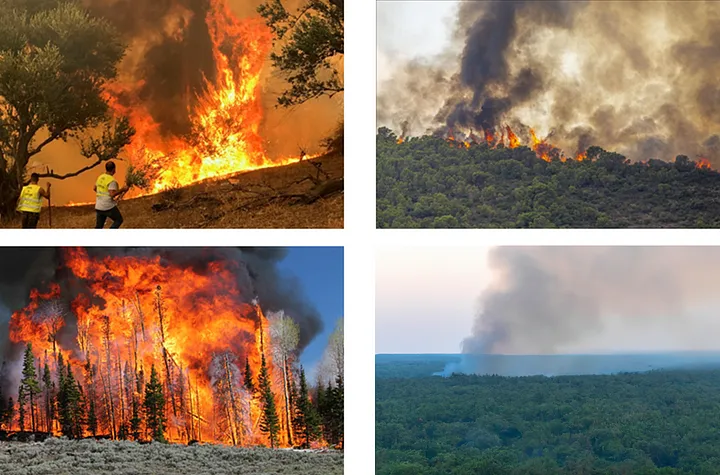
Another challenge arose when we could not deploy the planned local processing machines at the pilot site due to constraints at the tower site. The local processing unit had the critical task of analyzing the imagery using AI and generating the alerts. We were bound to use small form-factor computers instead. Thankfully, 4G broadband internet was available at the same site. We chose to use Jetson Nano instead, a small, powerful computer that lets you run edge AI applications. With minimal AI-based filtering on-site, the Jetson Nano will send the imagery to the high-end remote machine for further investigation and alert generation.
Final thoughts and what’s to come
As we move forward, we will be integrating the data from Fire Detection cameras, Weather Stations, IoT sensors, and third-party data (i.e., weather data) on a dashboard. At the same time, AI models and machine learning will be performed at the backend that feeds the information to the dashboard. Our assumptions will be tested on the bench and eventually on-site in the subsequent sprints. This is not just a test of the technology frontier but also the technology development approach.
The project’s scope is being developed and adapted in an agile manner as the project goes on. Learnings are being incorporated into the next sprint planning. We aim to deliver a working technology that the forest fire rangers will eventually adopt across the country and globally.
Driven by the philosophy of ‘Learning Without Borders,’ the project team at LUMS is motivated and hopeful that our learnings from this project will bring value to other third-world economies as cost-effective and scalable innovations.
This blog post compliments the reflections of WWF in engaging the community in the proposed early warning system, which can be found here.